Sensitivity Analysis Based on Physical Properties to Permeability Coefficient of Cohesive Soil Using Artificial Neural Network
DOI:
https://doi.org/10.25299/jgeet.2024.9.1.13536Keywords:
ANN, AI, Permeability, Soil Physical PropertiesAbstract
Permeability is the ability of a soil to allow liquids to pass through. Of course the soil has a physical characteristic that can be known by laboratory testing. This study aims to determine the physical properties that most affect the coefficient of cohesive soil permeability using the Artificial Neural Network (ANN) tool, the results obtained will later be matched with actual conditions according to the context of engineering geology. The research method begins with an influence or sensitivity analysis using ANN which will produce a correlation coefficient (R). Then, these results will be compared with the influence analysis based on the value of the coefficient of determination (R2). After that, accuracy and error tests will be carried out using the Mean Absolute Percentage Error (MAPE), the highest accuracy values is categorized as the most influential physical property of the 7 physical property parameters, namely liquid limit, plastic limit, plasticity index, %sand, %fines, %silt, and %clay. Based on the result of the analysis, %fines is the parameter that most influences permeability and is able to make very strong predictions with an R value using an ANN of 0.9941875, an R2 value of 0.6336, an accuracy of 99.6962%, and a MAPE of 0.3038%. These results are compared with the existing empirical equations with an accuracy of 96.4393% and MAPE of 3.5607%. It can be concluded that ANN is more effective and optimal in making predictions. In this case, in the context of engineering geology, the more %fines, the smaller the permeability coefficient of the soil.
Downloads
References
Abiodun, O. I., Jantan, A., Omolara, A. E., Dada, K. V., Mohamed, N. A. E., & Arshad, H. (2018). State-Of-The-Art In Artificial Neural Network Applications: A Survey. Heliyon, 4(11), e00938.
Amanda, N. (2022). Model Korelasi Empiris Sifat Fisik Dengan Permeabilitas Tanah Menggunakan Artificial Neural Network.
Das, B. M. (1995). Mekanika Tanah (Prinsip-prinsip Rekayasa Geoteknis). In Penerbit Erlangga. Erlangga.
Fernando, H., Nugroho, S. A., Suryanita, R., & Kikumoto, M. (2021). Prediction of SPT Value Based on CPT Data and Soil Properties Using ANN With and Without Data Normalization. International Journal of Artificial Intelligence Research, 5(2), 123–131.
Ibrahim, D. (2016). An Overview of Soft Computing. Procedia Computer Science, 102(August), 34–38.
Irawan, H., Nugroho, S. A., & Satibi, S. (2013). Korelasi Permeabilitas Berdasarkan Ukuran Butiran dan Plastisitas Tanah. 1989.
Kusuma, S., Suhery, C., & Hidayati, R. (2021). Implementasi Metode Weighted Moving Average Pada Sistem Prediksi Stok Tembakau Lokal Berbasis Web (Studi Kasus Outlet Progressive Nicotiana). Coding Jurnal Komputer Dan Aplikasi, 9(3), 400–410.
Long, M., & Boylan, N. (2021). In-Situ Testing of Peat - a Review and Update on Recent Developments. Geotechnical Engineering Journal of the SEAGS & AGSSEA, 43(4).
Maharani, P. H., Sunarminto, B. H., & Hanudin, E. (2015). Penggunaan Fungsi Pedotransfer untuk Memperkirakan Permeabilitas Tanah di Sumatera Selatan dan Riau. 18(1), 37–43.
Naim, Priadi, E., & Aprianto. (2019). Pemetaan Zonasi Geoteknik Di Kota Pontianak Berdasarkan Data Konsistensi dan Sifat-Sifat Tanah Dengan Sistem Informasi Geografis. Jurnal Agroekoteknologi Tropikapertanian, 24(1)(21), 16.
Nasution, H. (2020). Implementasi Logika Fuzzy pada Sistem Kecerdasan Buatan. ELKHA: Jurnal Teknik Elektro, 4(2), 4–8.
Padagi, E. A., Priadi, E., & Aprianto. (2015). Korelasi Nilai N-SPT Terhadap Sifat Fisik dan Mekanis Tanah. Jurnal Mahasiswa Teknik Sipil Universitas Tanjungpura, 1(1), 1–11.
Pratama, I. M. R., Yulianti, I., & Masturi, M. (2017). Analisis Sebaran Butiran Agregat Tanah, Sebaran Butir Primer Tanah, dan Permeabilitas Tanah Pada Pabrik Teh. JIPF (Jurnal Ilmu Pendidikan Fisika), 2(1), 7.
Raei, B., Ahmadi, A., Neyshaburi, M. R., Ghorbani, M. A., & Asadzadeh, F. (2021). Comparative Evaluation Of The Whale Optimization Algorithm And Backpropagation For Training Neural Networks To Model Soil Wind Erodibility. Arabian Journal of Geosciences, 14(1).
Rochmawati, R., Irianto, & Lau, L. M. (2020). Studi Eksperimental Penentuan Jenis Tanah Berdasarkan Distribusi Ukuran Butiran Tanah. Journal of Portal Civil Engineering, 3(2), 145–154.
Setiawan, B., Suryanita, R., & Djauhari, Z. (2017). Prediksi Tingkat Kinerja Struktur Gedung Kantor Berdasarkan Mutu Beton Dengan Metode Jaringan Saraf Tiruan. SIKLUS: Jurnal Teknik Sipil, 3(2), 107–116.
Soedarmo, G. D., & Punomo, S. J. E. (1993). Mekanika Tanah 1 (Vol. 15, Issue 2, pp. 1–23). KANISIUS.
Sugiyono. (2007). Metode Penelitian Kuantitatif Kualitatif dan R&D. Alfabeta.
Sutojo, T., Mulyanto, E., & Suhartono, V. (2011). Kecerdasan Buatan. ANDI.
Yantrapalli, S. K., P, H. K., & K, S. (2018). A Study On Influence Of Real Municipal Solid Waste Leachate On Properties Of Soils In Warangal, India. Journal of Geoscience, Engineering, Environment, and Technology, 3(1), 25.
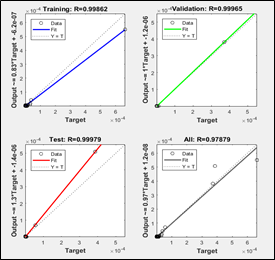
Downloads
Published
Issue
Section
License
Copyright (c) 2024 Journal of Geoscience, Engineering, Environment, and Technology

This work is licensed under a Creative Commons Attribution-ShareAlike 4.0 International License.
Copyright @2019. This is an open-access article distributed under the terms of the Creative Commons Attribution-ShareAlike 4.0 International License which permits unrestricted use, distribution, and reproduction in any medium. Copyrights of all materials published in JGEET are freely available without charge to users or / institution. Users are allowed to read, download, copy, distribute, search, or link to full-text articles in this journal without asking by giving appropriate credit, provide a link to the license, and indicate if changes were made. All of the remix, transform, or build upon the material must distribute the contributions under the same license as the original.