Classification and Distribution Of Mangrove Genus Using Multispectral Unmanned Aerial Vehicle (UAV) In The Waters Of Lancang Island, Kepulauan Seribu, Indonesia
DOI:
https://doi.org/10.25299/jgeet.2024.9.2.17195Keywords:
Lancang Island, Mangrove, UAV MultispectralAbstract
Mapping of mangrove distribution is important as basic information in mangrove resource management. development of remote sensing technology with multispectral unmanned aerial vehicle (UAV) with high spatial resolution. This study aims to determine the classification and distribution of mangrove genera using a pixel-based classification method and calculate the accuracy level of mangrove genus classification using a multispectral unmanned aerial vehicle (UAV) in Lancang Island Waters, Kepulauan Seribu. This research was carried out in August 2023 by obtaining 481 mangrove genus observation points using the DJI Phantom 4 multispectral drone. Image classification was processed using a pixel-based classification method with two classification levels, including level 1 (mangrove), resulting in an area of 18.72 ha. Level 2 (mangrove genus) uses guided classifications such as Support Vector Machine (SVM), K-Nearest Neighbor (KNN), and Random Forest (RF). Based on the classification results, the best results were obtained using the RF algorithm with an accuracy of 89.78% and a kappa index of 0.51, followed by the SVM algorithm with an accuracy of 89.78% and a kappa index of 0.45, then using the KNN algorithm with an accuracy of 88.32% and a kappa index of 0.43.
Downloads
References
Alfani, F., Mangrove Mapping on Lancang Island Using Drones and Sentinel-2A with Object Based Image Analysis and Pixel Based Analysis Methods.
Aslan, A., Rahman, A. F., Warren, M. W., & Robeson, S. M. (2016). Mapping spatial distribution and biomass of coastal wetland vegetation in Indonesian Papua by combining active and passive remotely sensed data. Remote Sensing of Environment, 183, 65–81.
Bengen, D.G. 2001. Technical Guidelines for the Introduction and Management of Mangrove Ecosystems, Center for Coastal and Marine Resources Studies. Bogor Agricultural University. Bogor.
Candra ID, Siregar VP, Agus SB. 2017. Geomorphological Zone and Benthic Habitat Mapping on Kotok Besar Island Using Object-Based Classification. Journal of Fisheries Technology and Fisheries. 8(2):209-219.
Cao, J., Leng, W., Liu, K., Liu, L., He, Z., & Zhu, Y. (2018). Object-based mangrove species classification using unmanned aerial vehicle hyperspectral images and digital surface models. Remote Sensing, 10(1), 89.
Giri C. Ochieng E. Tieszen LL. Zhu Z. Singh A. Loveland T. Masek J. Duke N. 2011. Status and distribution of mangrove forests of the world using earth observation satellite data. Glob. Ecol. Biogeogr. 20(1):154–159.
Hamad IY, Staehr PAU, Rasmussen MB, Sheikh M. 2022. Drone-Based Characterization of Seagrass Habitats in the Tropical Waters of Zanzibar. Remote Sensing. 14,680.
Hendrawan. 2018. Study of mangrove cover and density using satellite images on Sebatik Island, North Kalimantan [Thesis]. Bogor (ID): IPB Postgraduate Program.
Heumann BW. 2011. An object-based classification of mangroves using a hybrid decision tree-support vector machine approach. Remote Sens. 3(11): 2440- 2460.
Hogarth PJ. 2007. The Biology of Mangroves and Seagrasses. Third Edit. New York: Oxford University Press: Oxford. UK
Hruska, Ryan; Mitchell, Jessica; Anderson, Matthew; Glenn, Nancy F. (2012). Radiometric and Geometric Analysis of Hyperspectral Imagery Acquired from an Unmanned Aerial Vehicle. Remote Sensing, 4(9), 2736–2752.
Jenning SB, Brown ND dan Sheil D. 1999. Assessing forest canopies and understorey illumination: canopy closure, canopy cover and other measures. Forestry. 72(1): 59–74.
Jones TG. Glass L. Gandhi S. Ravaoarinorotsihoarana L. Carro A. Benson L. Ratsimba HR. Giri C. Randriamanatena D. Cripps G. 2016. Madagascar’s mangroves: Quantifying nation-wide and ecosystem specific dynamics. and detailed contemporary mapping of distinct ecosystems. Remote Sens. 8(2).
Kanekaputra, T. (2018). Mapping Of Mangrove Species Avicennia Sp. Based On Field Spectral Reflection And Worldview-2 Imagery (Doctoral Dissertation, Universitas Gadjah Mada).
Kelley, L. C., Pitcher, L., & Bacon, C. (2018). Using Google Earth engine to map complex shadegrown coffee landscapes in Northern Nicaragua. R
Kuenzer C. Bluemel A. Gebhardt S. Quoc TV. Dech S. 2011. Remote sensing of mangrove ecosystems: A review. Remote Sens. 3(5): 878-928.
Mallinis, G., I. Mitsopoulos, & I. Chrysafi. 2018. Evaluating and comparing Sentinel 2A and Landsat-8 Operational Land Imager (OLI) spectral indices for estimating fire severity in a Mediterranean pine ecosystem of Greece. GISci. Remote Sens., 55(1): 1–18.
Mastu LOK, Nababan B, Panjaitan JP. 2018. Object-Based Benthic Habitat Mapping using Sentinel-2 Imagery in the Waters of Wangi-Wangi Island, Wakatobi Regency. Journal of Tropical Marine Science and Technology. 10(2):381-396
Minister of the Environment. 2004. Decree of the Minister of Environment Number 201 of 2004 concerning Standard Criteria and Guidelines for Determining Mangrove Damage. Jakarta.
Mitchell, Jessica J.; Glenn, Nancy F.; Anderson, Matthew O.; Hruska, Ryan C.; Halford, Anne; Baun, Charlie; Nydegger, Nick (2012). [IEEE 2012 4th Workshop on Hyperspectral Image and Signal Processing: Evolution in Remote Sensing (WHISPERS) - Shanghai, China (2012.6.4-2012.6.7)] 2012 4th Workshop on Hyperspectral Image and Signal Processing (WHISPERS) - Unmanned aerial vehicle (UAV) hyperspectral remote sensing for dryland vegetation monitoring.
Murmu S, Biswas S. 2015. Application of fuzzy logic and neural network in crop classification: a review. Aquatic Pocedia. 4(1):1203-1210.
Nababan B, Mastu LOK, Idris NH, Panjaitan JP. 2021. Shallow-Water Benthic Habitat Mapping Using Drone with Object Based Image Analyses. Remote sensing. 13, 4452.
Nagarajan, P., Rajendran, L., Pillai, N. D., & Lakshmanan, G. (2022). Comparison of machine learning algorithms for mangrove species identification in Malad creek, Mumbai using WorldView-2 and Google Earth images. Journal of Coastal Conservation, 26(5), 44.
Oktorini, Y., Darlis, V. V., Wahidin, N., & Jhonnerie, R. (2021, March). The Use of SPOT 6 and RapidEye Imageries for Mangrove Mapping in the Kembung River, Bengkalis Island, Indonesia. In IOP Conference Series: Earth and Environmental Science (Vol. 695, No. 1, p. 012009). IOP Publishing.
Oleksyn S, Tesetto L, Raoult V, Joyce KE, Williamson JE, 2021. Going Batty: The Challenges and Opportunities of Using Drones to Monitor the Behaviour and Habitat Use of Rays. Drones. 5,12.
Osei Darko, Patrick, Margaret Kalacska, J. Pablo Arroyo-Mora, and Matthew E. Fagan. "Spectral complexity of hyperspectral images: a new approach for mangrove classification." Remote Sensing 13, no. 13 (2021): 2604. Ikonos quickbird
Pretzsch H, Biber P, Uhl E, Dahlhausen J, Rotzer T, Caldentey J, Koike T, Con TV, Chavanne A, Seifert T, Toit BD, Farnden C, Pauleit S. 2015. Crown Size and Growing Space Requirement of Common Tree Species in Urban Centres, Parks, and Forests. Urban Forestry & Urban Greening. 14(3): 466-479.
Rahmandhana, A.D., Aditama, M.H. And Mahendra, D., 2022. Utilization Of Gis Remote Sensing Technology For Pt Amman Mineral Nusa Tenggara's Watershed Reclamation And Rehabilitation Program. Proceedings Of The Perhapi Annual Professional Meeting, Pp.489-502.
Ridha, S. M. (2021). Mapping Mangrove Species Using Worldview-2 Imagery And Object-Based Classification In Clungup Mangrove Conservation Malang, East Java (Doctoral Dissertation, Universitas Gadjah Mada).
Rosmasita, R., Siregar, V. P., &; Agus, S. B. Classification of Mangroves Based on Objects and Pixels Using Sentinel-2b Imagery in Liong River, Bengkalis, Riau Province. Journal of Tropical Marine Science and Technology, 10(3), 601-615.
Sanjoto, T.B., Husna, V.N. and Sidiq, W.A.B.N., 2022. Spectral angle mapper algorithm for mangrove biodiversity mapping in Semarang, Indonesia.
Siregar, V. P., Agus, S. B., & Jhonnerie, R. (2019, May). An object-based classification of mangrove land cover using Support Vector Machine Algorithm. In IOP conference series: earth and environmental science (Vol. 284, No. 1, p. 012024). IOP Publishing.
Su, X., Wang, X., Song, D., Zhao, J., Fan, J., & Yang, Z. (2020, July). Improved Spectral Angle Mapper
applications for mangrove classification using SPOT5 imagery. In International Conference in Communications, Signal Processing, and Systems (pp. 1232-1243). Singapore: Springer Singapore.
Sukmawati, T. S. 2008. Detection of mangrove ecosystems in Cilacap, Central Java with Alos satellite imagery. Thesis. Marine Science and Technology Study Program, Faculty of Fisheries and Marine Sciences: Bogor Agricultural University.
Thampanya U, Vermaat JE, Sinsakul S, Panapitukkul N. 2006. Coastal erosion and mangrove progradation of Southern Thailand. Estuar Coast Shelf Sci. 68(1): 75-85
Tomlinson BP. 1986. The Botany of Mangroves Second Edition Mangroves. Cambridge University Press
Tridawati, A., Wikantika, K., Susantoro, T. M., Harto, A. B., Darmawan, S., Yayusman, L. F., & Ghazali, M. F. (2020). Mapping the distribution of coffee plantations from multiresolution, multi-temporal, and multi-sensor data using a random forest algorithm. Remote Sensing, 12(23), 3933.
ZABRINA, Waode Shifa. 2023. Analysis of Maximum Likelihood and Support Vector Machine Classification Methods on Mangrove Mapping.
Zhang X. Tian Q. 2013. A mangrove recognition index for remote sensing of mangrove forest from space. Curr. Sci. 105(8):1149–1154
Zhang X. Treitz PM. Chen D. Quan C. Shi L. Li X. 2017. Mapping mangrove forests using multi-tidal remotely-sensed data and a decision-tree-based procedure. Int J Appl Earth Obs Geoinf. 62(1):201–214.
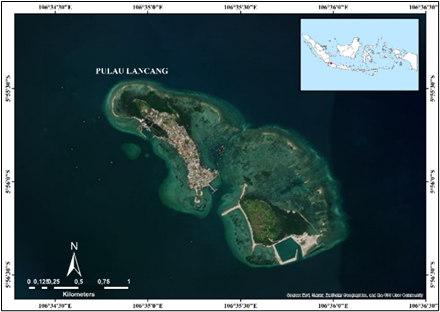
Downloads
Published
Issue
Section
License
Copyright (c) 2024 Journal of Geoscience, Engineering, Environment, and Technology

This work is licensed under a Creative Commons Attribution-ShareAlike 4.0 International License.
Copyright @2019. This is an open-access article distributed under the terms of the Creative Commons Attribution-ShareAlike 4.0 International License which permits unrestricted use, distribution, and reproduction in any medium. Copyrights of all materials published in JGEET are freely available without charge to users or / institution. Users are allowed to read, download, copy, distribute, search, or link to full-text articles in this journal without asking by giving appropriate credit, provide a link to the license, and indicate if changes were made. All of the remix, transform, or build upon the material must distribute the contributions under the same license as the original.